Differentiating your Business with Data Quality
In the retail industry, data quality is often overlooked as an aspect of running a business. But on the contrary, it is a competitive differentiator and is quickly gaining priority among businesses.

Correctly managing data is crucial.
It is important to note that random, unqualified, and unfiltered data can become counterproductive. This means decisions will be made based on incorrect and improper data, which may result in wasting time and resources.
On the flip side, good-quality data ensures an informed decision-making process and provides the right insights. However, ensuring quality data can be challenging if not presented with the right tools. Sure, structured datasets are essential, but what really matters is how relevant your data is to your solution; the better the quality, the more a business can get from it.
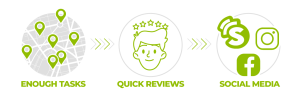
Ensuring Data Quality while crowdsourcing
Crowdsourcing techniques help significantly when gathering data, as large quantities are usually needed in real-time. Here’s how to always guarantee a plausible outcome when crowdsourcing high-quality data:
1. Avoid unambiguous guidelines
Crowdsourcing implies enlisting the services of many people over the internet to contribute relevant information to your requirements. Thus, it is imperative to present detailed instructions on what you need them to do, as providing ambiguous requirements will surely lead the crowd to fail to provide correct and relevant details.
However, to avoid this, you need only present clear guidelines on what you need from them, how you need the task to be performed, how long it can take, and more. Include example images that help them follow the instructions better. Moreover, the guidelines should be kept clear and concise for a better outcome.
2. Ensure data diversity and avoid bias
Data bias can negatively impact the accuracy of the information you present. It occurs when, for example, a significant volume of data is prone to lean toward a particular factor, such as race, gender, age, and so on. Therefore, to reduce biased results when crowdsourcing, ensuring that the crowd is as diverse as possible is crucial.
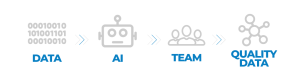
3. Multiple Quality Assurance Processes
Ideally, a QA needs to involve at least two processes:
Artificial Intelligence is when the information can be entered into a database and easily organized and filtered automatically.
A process led by a qualified in-house team to review the information, assuring quality. Here, the team reviews if all the required fields are filled, the data is well presented, and there are no errors in submitting tasks.
Lucky for you, SmartSpotter has a platform that guarantees actionable real-time insights with quality data, presented in an easy-to-understand dashboard with analytics that explain what it all means.
That way, you can target effective, data-driven action to ensure retailer-supplier agreements are fulfilled.